Automatic analysis of mammograms
- Department BAMJO
- Fields involved Image analysis
We develop methods for image analysis of mammograms in close collaboration with the Cancer Registry of Norway and various breast diagnostic centres around the country.
We have extensive experience in this domain, and were one of the first institutes to apply deep learning techniques for this purpose. Our partnership with the Cancer Registry and regional diagnostic centres has enabled access to millions of mammograms labelled with confirmed cancer diagnoses, sourced from the national screening programme.
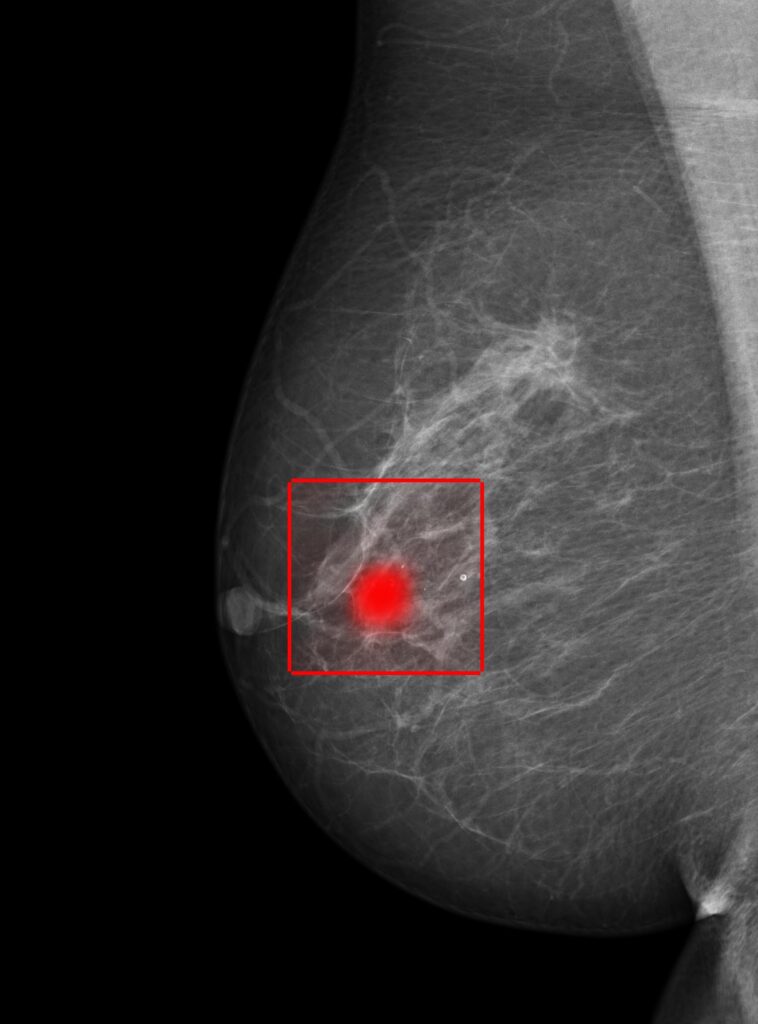
Partner: The Cancer Registry of Norway
Further reading:
Visual Intelligence – visual-intelligence.no
BreastScreen Norway – kreftregisteret.no
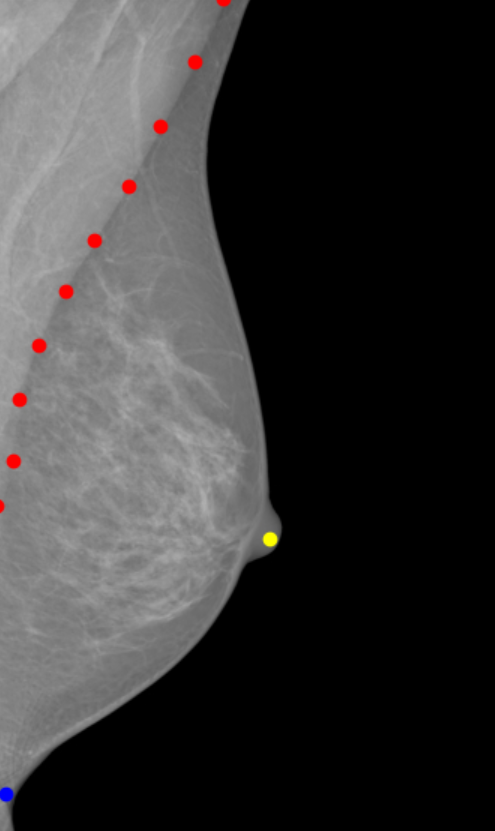
Explainable artificial intelligence (XAI)
Our models are at the forefront of breast cancer detection, surpassing academic and commerical counterparts. To ensure practical application and trustworthiness, it is important that humans are able to understand how the models make decisions. To that end, we use XAI. These techniques can be used to generate heatmaps that highlight which parts of the breast contribute to the model’s prediction. We also integrate XAI in the model itself in order to automatically identify the area of the breast that warrants an additional analysis in higher resolution by a second model.
Adaptable models for different datasets
A key feature of cancer is the uncontrolled growth and spread of abnormal cells. We are developing deep learning models that can extract information from earlier mammograms in order to detect changes that may be malignant. Another important issue is the models’ ability to generalise effectively across diverse datasets, including different populations, time periods and imaging equipment. Consequently, we focus on exploring methods that enable the models to adapt to various settings without needing to be retrained for each new domain.
Improving mammographic image quality
Our primary focus is cancer detection, but good image quality is a vital component in this process, and we are therefore developing methods to assist and improve analysis and image quality. Key factors to image quality are image blur, tissue density and breast positioning within the images. Presently, we are looking into the latter, breast positioning, and developing deep learning graph convolution models that can identify significant areas in the breasts. These models can aid radiographers in assessing mammogram quality, and are able to provide immediate feedback during imaging, for instance if key points are missing or a breast is positioned incorrectly.