Advancing marine services with computer vision
- Department BAMJO
- Fields involved Image analysis, Marine image analysis
- Industries involved Ocean
Fisheries and aquaculture are major industries in Norway, and marine image data are acquired in different formats for a wide range of tasks. Together with our project partners, we have developed automatic solutions for marine image analysis that enhance knowledge and efficiency in the marine sector, and also enable continuous monitoring of ecosystems to ensure sustainable fisheries and harvest quotas.
Automatic extraction of information from marine image data
Today, vast amounts of marine data are being collected, encompassing optical images, videos, and acoustic surveys. These extensive datasets contain information that is crucial for sustainable fisheries and resource management. As marine services shift towards real-time analysis, data volumes are anticipated to surge. Manual processes are not equipped to handle such volumes. Our objective has been to develop automatic solutions that are capable of extracting valuable information from these complex images. This will not only enhance efficiency and accuracy, but also drive advancements in both marine science and deep learning.
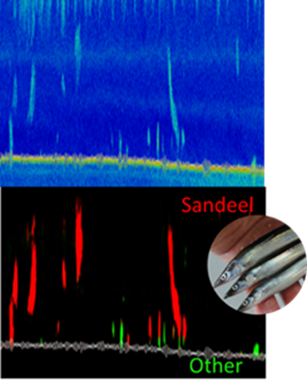
Using deep learning to detect acoustic targets
When it comes to acoustical data, our goal is to automatically estimate fish quantities and identify their species for abundance estimation. We have achieved this through a deep learning-based method for detecting acoustic targets, placing special emphasis on handling data variations across surveys. Methods have been further enhanced by incorporating contextual information like depth and distance to the seabed.
Automated age estimation and transparent AI
The Institute of Marine Research (IMR) has developed methods for automated age estimation of Greenland halibut using otolith images. Parallel to this pioneering work, NR has explored Explainable Artificial Intelligence (XAI) methods to understand how the network interprets these images. Our study revealed that deep learning techniques interpret images differently than humans. We also observed reduced performance with otolith data from other laboratories than those used for training. To address this, we have developed methods that adapt the model trained on Norwegian otolith images to perform well on otolith images from other labs. Our results can be accessed on the DeepOtolith web portal.
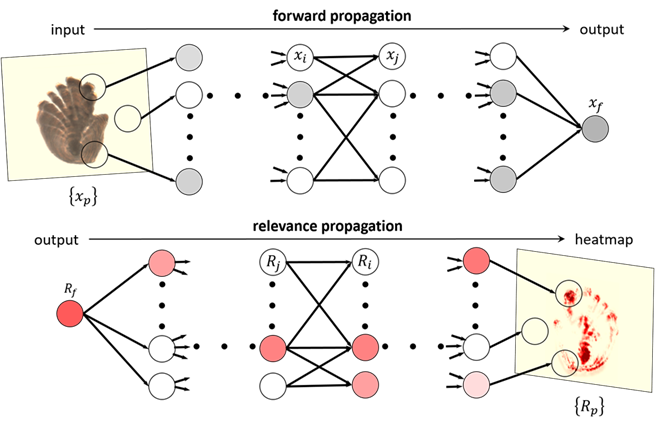
Project: COGMAR
Partners: The Institute of Marine Research (IMR), UiT The Arctic University of Norway and DeepVision
Funding: The Research Council of Norway
Period: 2017-2022
Resources
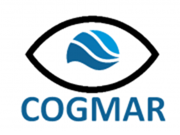