Deep learning for seismic data (DELI)
- Department BAMJO
- Fields involved Image analysis, Machine learning
- Industries involved Natural resources
We are developing advanced tools geologists can generate interpretations much faster than before, while also achieving more comprehensive insights about the subsurface.
In this project we develope deep learning-based methods for interpreting seismic data in collaboration with Equinor. Our methods are based on convolutional networks trained on geologists’ interpretations of the seismic data.
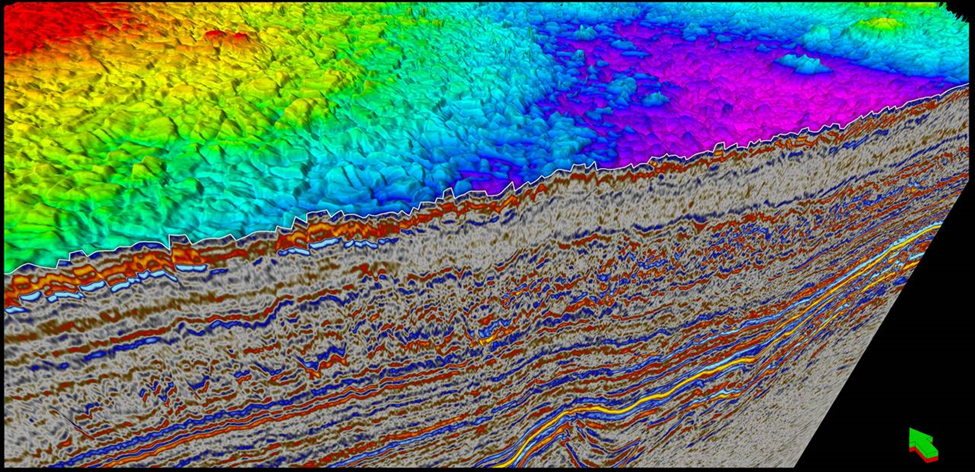
Creating geological images of the subsurface
Energy companies gather extensive seismic data when searching for hydrocarbons. In Norway, where oil and gas fields are offshore, seismic ships are employed to collect this data. To map and understand the subsurface, these vessels use air guns to transmit sound waves through the water and into the sediment below. The resulting seismic reflections create detailed geological images of the subsurface.
Increasing efficiency in seismic data analysis
A seismic interpreter analyses the data to map areas for potential prospects and hydrocarbon traps. The interpretation provides insight into sediment deposition and conditions, tectonic events, hydrocarbon formation, migration, and trappings. Automatic techniques can be used for clear, continuous reflections, but manual interpretations by geologists or geophysicists are usually necessary. However, manual interpretations are time-consuming, and due to the enormous volume, only 3-4% of the data are typically used in the process. We are working with Equinor to address these issues by creating tools that reduce interpretation time, sometimes from weeks to hours.
Challenges with deep learning for seismic data
Applying deep learning to seismic data presents numerous challenges. The data is complex, non-standard image data represented as three-dimensional cubes. Moreover, the models are trained on the geologists’ interpretations, which may contain inconsistencies, noise and errors. While these factors are often inconsequential in manual interpretations, they can significantly affect the effectiveness of machine learning. Through DELI, we have developed a solution capable of handling these challenges which accurately predicts high-quality surfaces despite of the complexity of the task.
To learn more about this project, please contact:
Project: Deep Learning Seismic Interpretation (DELI)
Partner: Equinor
Funding: Equinor
Period: 2017-