Marine image analysis
- Department BAMJO
- Fields involved Image analysis
Every day, vast amounts of observational data are generated for monitoring and resource management in the marine sector. These data contain valuable information critical for sustainable fisheries and aquaculture, but manual methods are insufficient to handle the volume and complexity. At NR, we develop deep learning-based methods that automate the collection, detection and classification of data, enabling efficient processes that are precise and scalable.
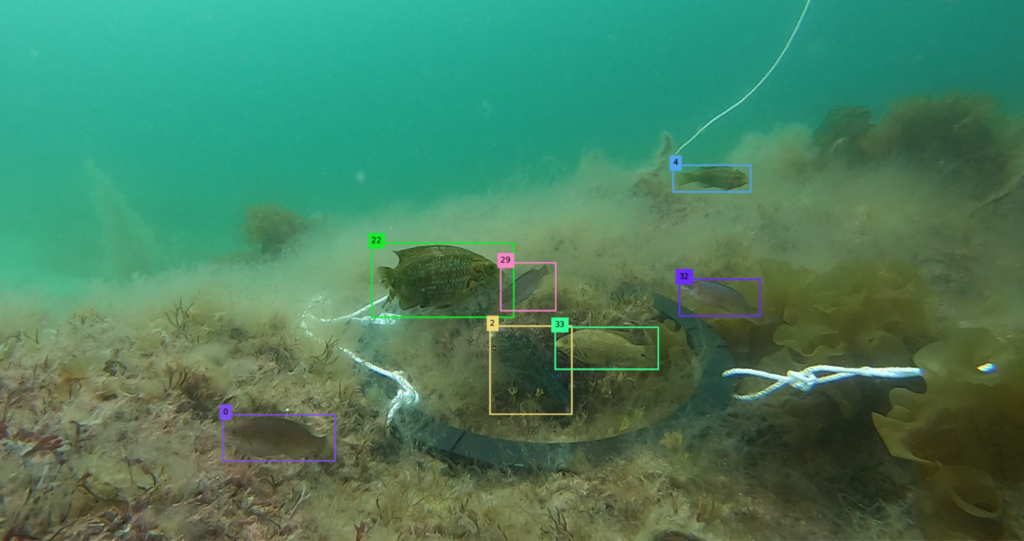
Efficient analysis of complex marine data
Vast amounts of complex observation data are being collected in the marine sector. These data contain valuable information critical for monitoring marine stocks, ecosystems, and ensuring sustainable fisheries and harvest. The sources are diverse, ranging from optical imagery and videos, to acoustic surveys. Next generation marine services, like real-time analysis of trawl content, will further increase the amount of data.
As the volume continues to grow, manual analysis is increasingly inefficient. Advancements in deep learning provide solutions to address these issues. At NR, we use deep learning to develop methods for automatic analysis and extraction of various types of marine image data, such as underwater videos and images, sonar acoustics, microscopic images of otoliths, and drone images of marine mammals.
Automated tracking of fish populations
Underwater cameras are increasingly used to closely monitor fish populations. They may be mounted in trawls, fish pens or near underwater habitats. Analysing the data from these cameras manually is costly, time-consuming, and ineffectual. However, by utilising deep learning algorithms, fish in these videos can be automatically located, classified and tracked over time. This can enhance the efficiency and effectiveness of marine monitoring and management.
Detection and classification of data
The complexity of some types of marine image data and scarcity of training data present significant challenges. Acoustic trawl surveys are, for example, not typically labelled with high levels of detail (i.e. strong labels). We developed a method for detecting and classifying schools of sandeels based on strongly labelled acoustic data and are currently adapting this method for weakly labelled acoustic data.
In the marine sector, it is common to leverage data from different sources in order to make final predictions. Because of this, we have expanded our sandeel model to include auxiliary data with a different modality as input. This has enhanced the model’s performance.
Transparent predictions with explainable artificial intelligence
When predictions are intended, such as model input for abundance estimation, trustworthiness is vital, and transparency and reliability are key.
Among our research areas, we are have examined how explainable artificial intelligence (XAI) can be used to understand what parts of otoliths our models focus on when making fish age predictions.
In the interest of reliability, we have also researched how the models perform on new datasets with slightly different distributions. For otoliths, this may happen when images come from different labs. Here, we are looking into methods for domain adaptation that, when applied to the otolith case, helps an existing model trained in one lab, to perform equally well for a different lab without retraining with labelled samples.
Selected projects
To learn more about marine image analysis at NR, please contact:
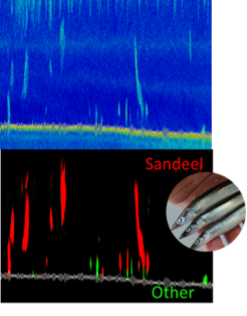
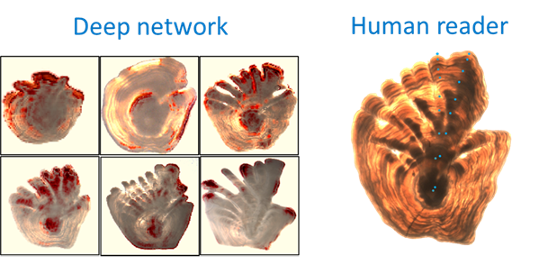