Automating railway inspections (AutoKontroll)
- Department BAMJO
- Fields involved Image analysis
- Industries involved Community
AutoKontroll aims to improve and automate essential railway inspection routines in Norway. By combining advanced artificial intelligence methods with innovative camera technology, we strive to enhance efficiency and reduce costs associated with inspections. This will contribute to greater safety and increased uptime for rail traffic, with the potential to transform railway operations across Norway. The project is a collaboration between NR and Bane NOR.
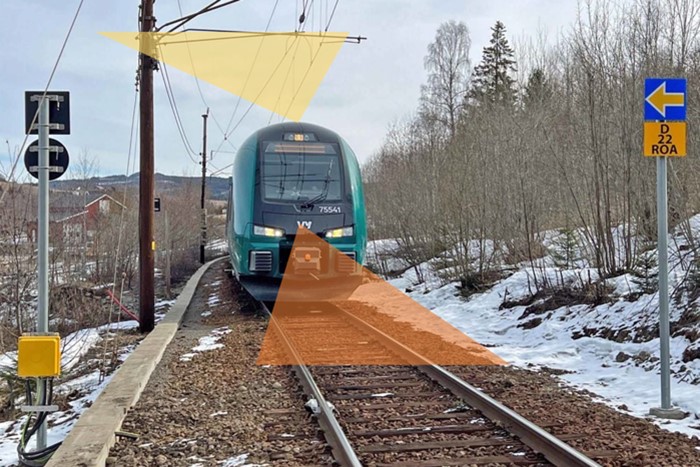
From manual to automated inspections
Current inspection routines are carried out manually by inspectors walking along the tracks to identify faults and defects. These inspections, often performed at night, are both time-consuming and costly. Our goal is to develop fully and partially automated solutions that utilise images captured by train-mounted cameras to detect faults and anomalies. This approach will enable more precise, regular and efficient inspections, significantly reducing the need for extensive manual labor.
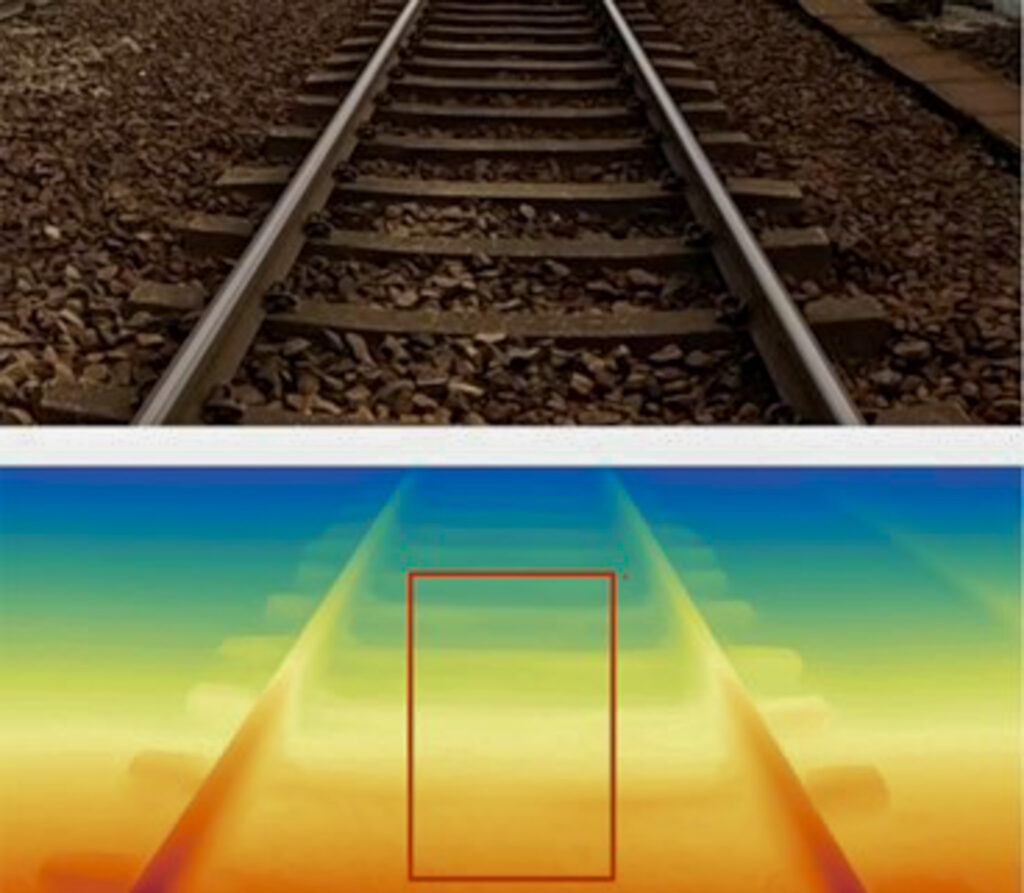
Image analysis with deep learning
In AutoKontroll, we are utilising a new and cost-efficient camera system that can be mounted on trains to capture images of railway infrastructure during every operation. These images are then analysed using deep learning techniques, including classification, semantic segmentation, object detection, self-supervised representation learning, and anomaly detection. These methods enable us to accurately identify faults and track changes over time.
Repeated imaging of the infrastructure provides valuable insights into how the condition of the railway evolves. This enables us to predict where and when potential faults may occur, detect anomalies early, monitor the progression of emerging issues, and initiate maintenance work before damage becomes critical.
Methods for standardisation and anomaly detection
The project presents several technical challenges, including developing a camera system capable of capturing standardised, high-quality images of railway infrastructure. Additionally, methods must be developed to compare images of the same components taken over time. Another key challenge lies in creating automated techniques for detecting relevant changes in image time series, as well as accurately identifying faults and anomalies.
The final results will be evaluated through a quantitative comparison between manual and automated inspections. This evaluation will provide a clear picture of the impact of automation and pinpoint areas where further improvement can be made.
To learn more about this project, please contact:
Project: AutoKontroll – Automated control of railways
Partner: Bane NOR
Funding: The Research Council of Norway
Period: 2024-2027
Other resources: