Intelligent cardiac ultrasounds
- Department BAMJO
- Fields involved Image analysis, Machine learning
- Industries involved Health
We have developed automated methods for cardiac ultrasound analysis. Our methods use deep neural networks to recognise objects and phenomena in ultrasound images and are guided by doctors’ annotations.
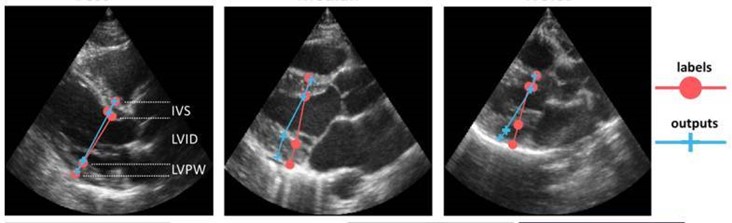
Cardiac ultrasounds are vital for assessing heart health. This procedure involves placing an ultrasound probe on the chest and directing it towards the heart. Sounds pulses from the probe are reflected from the organ structure, and the reflected signal thus provides an image of the heart. These images enable physicians to analyse various characteristics, such as distances between structures, valve function, patterns in ventricular wall motion, and blood flow.
Examinations are often time-consuming due to the need for manual adjustments and settings on the scanner for each patient, on top of the procedure itself. This leaves limited time for image interpretation and diagnosis, an issue cardiologists have expressed a need to simplify and resolve.
GE Vingmed Ultrasound develops scanners for ultrasound examinations of the heart. In collaboration with GE Vingmed and the University of Oslo, NR is developing machine learning-based tools and methods that will contribute to automating examination processes.
Our methods use deep neural networks to recognise objects and phenomena in ultrasound images, guided by doctors’ annotations. However, there are several challenges that require smart solutions. Ultrasound images are often noisy and indistinct, necessitating expert knowledge for correct annotation and access to sufficient training data. There is also a need for fast and compact algorithms that can be implemented directly into the scanners, yielding rapid results.
Through the INCUS project (2016-2019), NR and GE Vingmed successfully automated tasks for detecting the heart’s view angle, selecting appropriate Doppler measurements, and measuring distances within images. Several of our solutions have been integrated into Vingmed ultrasound scanners and are now in clinical use.
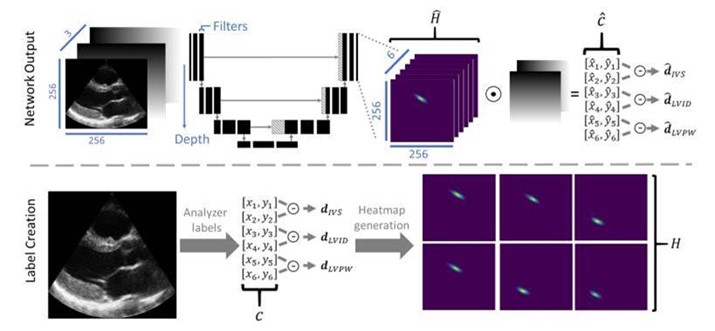
Project: INCUS; EchoAI
Partners: GE Vingmed Ultrasound and the University of Oslo (UiO)
Funding: GE Vingmed Ultrasound and the Research Council of Norway
Period: 2016-2019; 2020-2023
Further reading:
Gilbert, A., Holden, M., Eikvil, L., Aase, S. A., Samset, E., & McLeod, K. (2019). Automated left ventricle dimension measurement in 2D cardiac ultrasound via an anatomically meaningful CNN approach. I Q. Wang, A. Gomez, J Hutter, K. McLeod, V. Zimmer, O. Zettinig, R. Licandro, E. Robinson, D. Christiaens, E. Abaci Turk & A. Melbourne (Eds.), Smart Ultrasound Imaging and Perinatal, Preterm and Paediatric Image Analysis (1st ed., pp. 29-37). Springer. DOI: 10.1007/978-3-030-32875-7.