Estimating vegetation height across Africa
- Department BAMJO
- Fields involved Earth observation
- Industries involved Climate and environment
We have developed a deep learning-based value chain for multi-sensor satellite data. The value chain can be used to estimate average vegetation height and surface reflectance across large areas.
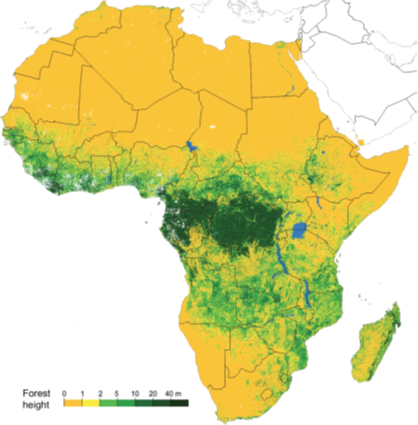
Advancing Earth observation with deep learning
In the past five years, significant advancements in artificial intelligence (AI) and deep learning have revolutionised the landscape of data analysis. The European Space Agency (ESA) launched the AI4EO initiative to target necessary steps to harness the full potential of AI for Earth observation (EO) applications across Europe.
During the project period, we addressed the needs outlined in the the AI4EO programme by developing a deep learning-based value chain for multi-sensor Sentinel-1 and -2 data. In this new approach, task specificity determines the required data, annotation type and choice of deep networks. We then use the data to train the network parameters. Notably, the network architectures primarily rely on the tasks, with data playing a secondary role. Data and network dependencies are encoded in the parameters undergoing training.
A flexible value chain for Earth observation
Our main focus areas were:
- Developing a new value chain for Earth observation: This new value chain is generic, flexible and easily adaptable to various applications. It is data driven, thus requiring training data to interpret Sentinel data for specific purposes.
- Using the value chain to estimate average vegetation height: We used transects of lidar data across forested regions in Liwale, Tanzania, to calculate the average vegetation height for a spatial grid. We then trained the deep learning-based value chain to predict the average vegetation height using Sentinel data. This was subsequently employed to generate a map depicting average vegetation heights for both the Liwale test data and the entire African continent.
- Using the value chain to estimate surface reflectance: To demonstrate the versatility and flexibility of the new value chain, we applied it to atmospheric correction of Sentinel-2. Specifically, we estimated surface reflectance (level 2A) from top-of-the-atmosphere (level 1C) Sentinel-2 data in southern Norway.
The use cases demonstrate the effectiveness of AI techniques, showcasing their predictive power and their ability to adapt to new tasks.
Project: The New Generation Value Chain for Earth Observation (NGVEO)
Partners: Phi Lab, ESA.
Funding: ESA
Period: 2018-2019
Further reading
Waldeland, A. U., Trier, Ø. D., & Salberg, A. B. (2022). Forest mapping and monitoring in Africa using Sentinel-2 data and deep learning. International Journal of Applied Earth Observations and Geoinformation, 111, 1-13. DOI: 10.1016/j.jag.2022.102840
Resources