Anomaly detection in wind and hydropower plants
- Department SAMBA
- Fields involved Machine learning, Statistical modelling
- Industries involved Climate and environment
Wind and hydropower plants can become more efficient by minimizing downtime due to wear and maintenance.

In this project we are developing methods for condition monitoring of hydro and wind turbines, based on sensors that measure temperature, pressure, and vibration, among other things. This way, serious and costly faults in the facilities can be detected before they occur and corrected within weeks rather than years.
Hydro and wind power plants can become more efficient by minimizing downtime due to wear and maintenance (Photo: Harald Danielsen).
The anomaly detection method consists of two basic steps:
- A prediction model for how the sensor measurements should behave given information about how the turbine is operated at any given time (e.g., the amount of guide vane opening).
- A model for detecting deviations from the estimated normal behavior.
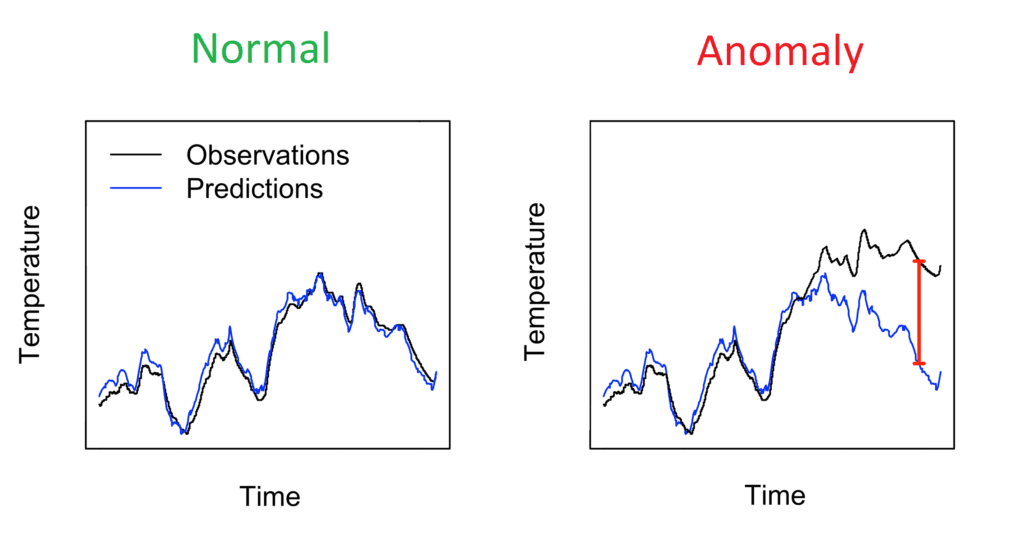
Anomalies are detected as large differences between predicted and observed sensor measurements.
Calibrating the method to detect only what is interesting is a central research challenge. If the operators of the power plant receive too many false alarms, they will lose trust in the system, and it becomes practically worthless.
It is also crucial that the operators can understand why an alarm has occurred, without being experts in machine learning or statistics.
Name: Anomaly detection in wind and hydropower plants
Partner: Statkraft
Period: 2022 – 2023