Mapping and map revision
- Department BAMJO
- Fields involved Earth observation
- Industries involved Climate and environment
We specialise in the research and development of algorithms specifically designed for mapping and map revision. Drawing on data collected from satellites, aircraft and drones, we are able to develop tailored methods that are applicable across various domains, including infrastructure, climate and even archaeology.
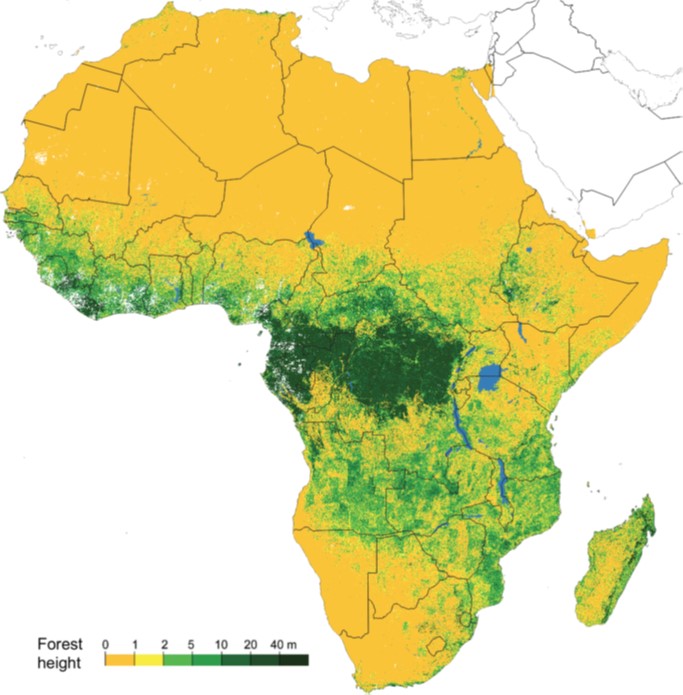
A map is a visual representation that highlights relationships among spatial elements like objects, regions, terrains and themes. Maps simplify information about the world and play a central role in conveying data at different levels, from overarching information at national level, all the way down to structures, roads and other features at local level.
Maps are crucial to a country’s security, socioeconomic development and environmental sustainability. National maps include topographic maps, cadastral maps and various thematic maps like landcover, population density and climate. Urban landscapes are constantly evolving, and frequent map revision is needed for effective urban management. Updated maps are used to inform decisions on urban planning, building infrastructure, managing emergencies and overseeing real estate. They are also used to monitor green spaces and address environmental issues in densely populated areas.
In Norway, mapping and map revision is managed collaboratively between the Norwegian Mapping Authority (Kartverket) and local municipalities, with active participation from various public and private entities, including NR.
Extensive experience in mapping methodologies
We have been developing mapping methodologies for more than 40 years, and began our work with thematic mapping using Landsat and SPOT satellites in the 1980s. In the 1990s, we started using digitalised aerial images to achieve high-resolution forest mapping, and we used data from the European Space Agency (ESA) to test airborne hyperspectral sensors, also known as imaging spectrometers, and synthetic aperture radars (SAR). Today, digital aerial imagery is standard, while imaging spectrometers are used on a case-by-case basis.
For large-scale mapping, different satellite sensors are available, from very-high resolution sensors on commercial satellites, to high-resolution sensors like MSI aboard Sentinel-2, and sensors with moderate resolution based on Sentinel-3 and similar satellites, which are used for daily, global mapping.
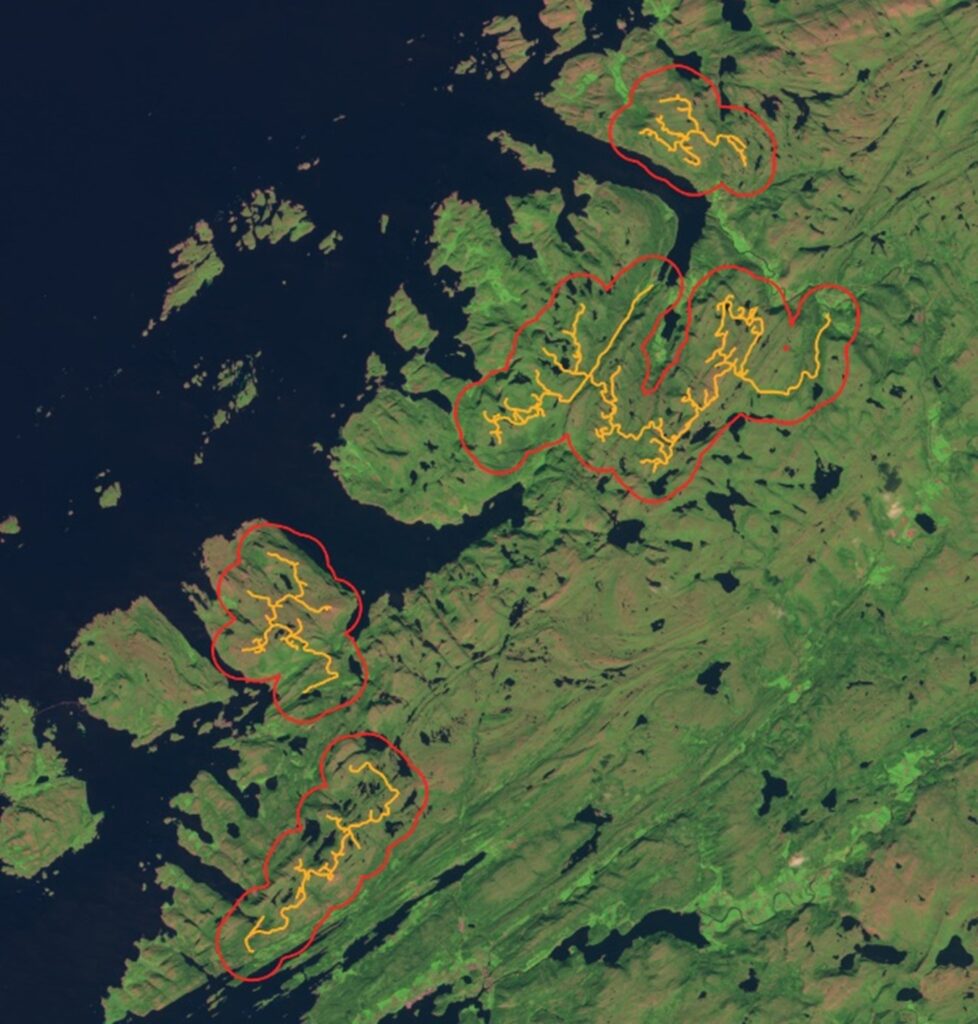
Automated methods for mapping and map revision
In several of our projects, NR is developing automated methods for mapping and map revision, including mapping vegetation height across the entire African continent, identifying new forest roads, detecting cultural heritage sites, classifying tree species, tracking building changes, and estimating birch pollen release.
The type of remote sensing data selected is determined by four factors:
- The size or scale of objects or phenomena, which dictates what resolution or grid cell size is required
- The area size
- The visibility of the objects or phenomena in the image
- The budget for data acquisition.
Typically, a compromise is made between these factors. For instance, when dealing with a vast area, using one image may result in a larger grid cell size. On the other hand, retrieving multiple images can be necessary in order to cover the area in sufficient detail.
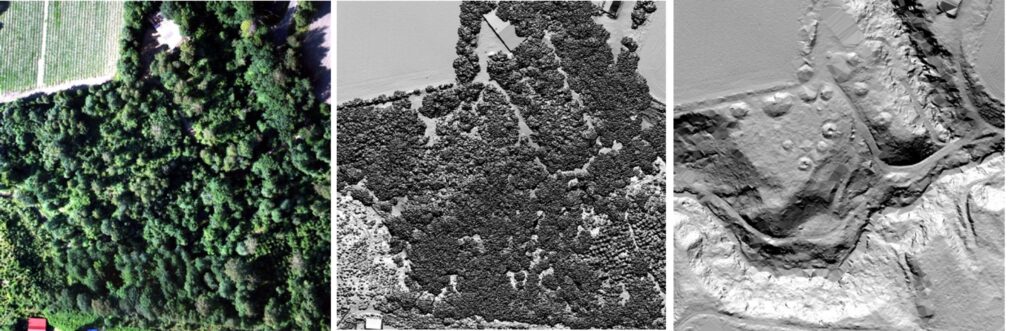
Automated mapping with deep learning
Automated mapping methods rely on artificial intelligence. This can be achieved by training deep neural networks with large amounts of labelled training data, or by utilising conventional methods if a well-established approach already exists. This can for example apply to assessments in land cover and land surface temperature.
Partners and clients:
- Bærum municipality
- The Directorate for Cultural Heritage (Riksantikvaren)
- The European Space Agency (ESA)
- Field Group
- The Norwegian Asthma and Allergy Association (NAAF)
- The Norwegian Institute of Air Research (NILU)
- The Norwegian Institute of Bioeconomy Research (NIBIO)
- The Norwegian Mapping Authority (Kartverket)
- The Norwegian Space Agency (NOSA)
- The Norwegian University of Life Sciences (NMBU)
- The Research Council of Norway
Further reading:
Jensen, A.C. (2024). Beyond output-mask comparison: A self-supervised inspired object scoring system for building change detection. Proceedings of the 5th Northern Lights Deep Learning Conference (NLDL), Proceedings of Machine Learning Research, 233, 97-103. https://proceedings.mlr.press/v233/jensen24a.html
Trier, Ø. D., Cowley, D. C., & Waldeland, A. U. (2018). Using deep neural networks on airborne laser scanning data: Results from a case study of semi-automatic mapping of archaeological topography on Arran, Scotland. Archaeological Prospection, 26(2), 165-175.
Trier, Ø. D., Reksten, J. H., & Løseth, K. (2021). Automated mapping of cultural heritage in Norway from airborne lidar data using faster R-CNN. International Journal of Applied Earth Observations and Geoinformation, 95, Article 102241. https://doi.org/10.1016/j.jag.2020.102241
Trier, Ø. D., Salberg, A.-B., Larsen, R., & Nyvoll, O. T. (2022). Detection of forest roads in Sentinel-2 images using U-Net. Proceedings of the Northern Lights Deep Learning Conference 2022, 3. https://doi.org/10.7557/18.6246
Waldeland, A. U., Trier, Ø. D., & Salberg, A.-B. (2022). Forest mapping and monitoring in Africa using Sentinel-2 data and deep learning. International Journal of Applied Earth Observation and Geoinformation, 111, Article 102840. https://doi.org/10.1016/j.jag.2022.102840