Snow observations for climate monitoring (Snow CCI)
- Department BAMJO
- Fields involved Earth observation, Snow monitoring, Climate and Environment
- Industries involved Climate and environment
The European Space Agency (ESA) Snow Climate Change Initiative (CCI) aims to advance our knowledge of snow in the climate system by generating consistent, high-quality, long-term snow maps. At NR, we are developing fractional snow cover products and deep learning-based algorithms that will differentiate between clouds and snow.
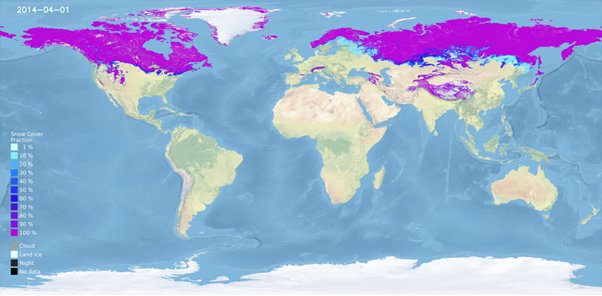
Seasonal snow cover is an important part of the Earth’s climate system. As the largest single component of the cryosphere, at its peak, seasonal snow cover accounts for about half of the land surface in the Northern hemisphere. Its range widely fluctuates between seasons, varying from around 2 million km2 in August to approximately 45 million km2 in January. Studying seasonal snow cover is crucial for climate analysis and modelling due to its impact on energy balance, the water cycle and availability of freshwater in mountain regions.
Reliable, high-quality snow maps over time
ESA Snow CCI is dedicated to generating consistent, high-quality, long-term snow maps that fulfill the standards set by the Global Climate Observing System (GCOS). The project’s primary objective is to generate consistent, long-term time series of daily global snow extent maps using optical satellite data, along with daily global snow water products from passive microwave satellite data.
Extensive experience with fractional snow cover products
NR plays several important roles in the project. We lead the work package for snow product generation, and we are responsible for various types of product development. We have extensive experience in the domain, having previously created snow cover fraction products for two ESA heritage sensors, which both provided one km resolution data which resulted in a daily snow product time series from 1995 to 2012.
We are also leveraging our heritage in Cryoclim, where we developed a full fractional snow cover product which generated a time series for the entire period between 1982 and 2020. This product employs a multi-sensor/multi-temporal analysis, incorporating a hidden Markov model (HMM) approach developed by NR. Our approach combines optical AVHRR sensors with passive microwave sensors SMMR, SSM/I and SSMIS. In addition, there is another layer of data that estimates the uncertainty of each measurement at the pixel level.
An algorithm for cloud-screening
Cloud-screening has been a persistent challenge in optical snow mapping since the advent of satellite remote sensing began. Clouds and snow share similar spectral properties, which can vary over time and with local conditions. We are developing a cloud-screening algorithm based on deep learning to distinguish between clouds and snow. If successful, our aim is to replace the algorithms used in current optical snow products with deep learning-based ones.
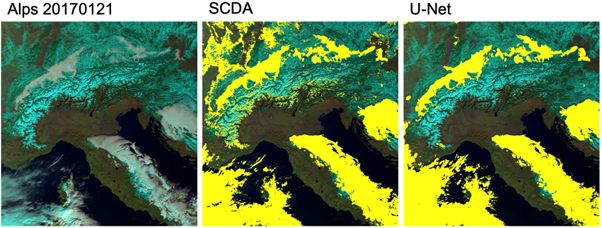
To learn more about this project, please contact:
Project: Snow Climate Change Initiative (Snow CCI)
Partners: ENVEO IT GmbH, Environment and Climate Change Canada (ECCC), EURAC Research, the Finnish Meteorological Institute (FMI), GAMMA Remote Sensing AG, the University of Berne (UBE) and the Finnish Environment Institute (SYKE)
Funding: The European Space Agency (ESA)
Period: 2018-2025