Automated analysis of drone data (SeaBee)
- Department BAMJO
- Fields involved Earth observation, Climate and Environment
- Industries involved Ocean, Climate and environment, Technology and industry
SeaBee is a Norwegian infrastructure project for drone-based research services for coastal and aquatic studies. It facilitates research, mapping and monitoring of habitats, animal communities and human-made impacts in these environments.
AI-based tools for easy and reliable analysis
The project aims to streamline data collection and analysis using aerial drones. Automating these processes will ensure cost-effective, high-quality data management, and offer a cutting-edge tool to address ongoing environmental challenges.
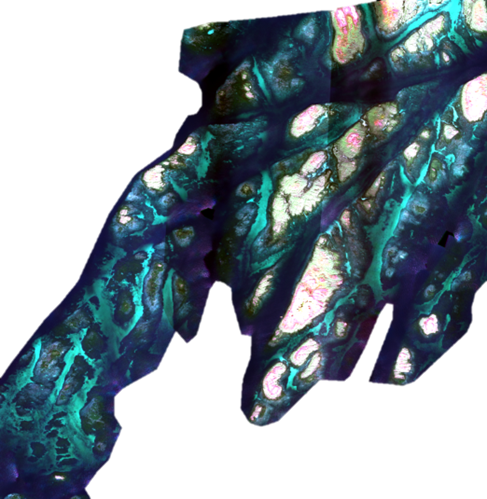
SeaBee provides:
- drones, equipment, and personnel for data collection,
- AI-based tools that enable easy and reliable image analysis,
- cloud-based storage and visualisation tools for easy access and inspection of drone images and analysis products.
Object detection and thematic mapping
SeaBee supports all kinds of image data, including multi-spectral, hyper-spectral and colour images (RGB). NR heads the development of AI algorithms, specifically deep learning, that enables automatic analysis of the data.
Our AI pipeline supports two main tasks: object detection and pixel-wise thematic mapping. Using advanced deep learning algorithms, the framework can be adapted to solve specific tasks, offering a large degree of flexibility with accurate results. Typical outputs include maps of marine habitats in coastal areas and automated recognition of marine mammals and sea birds.
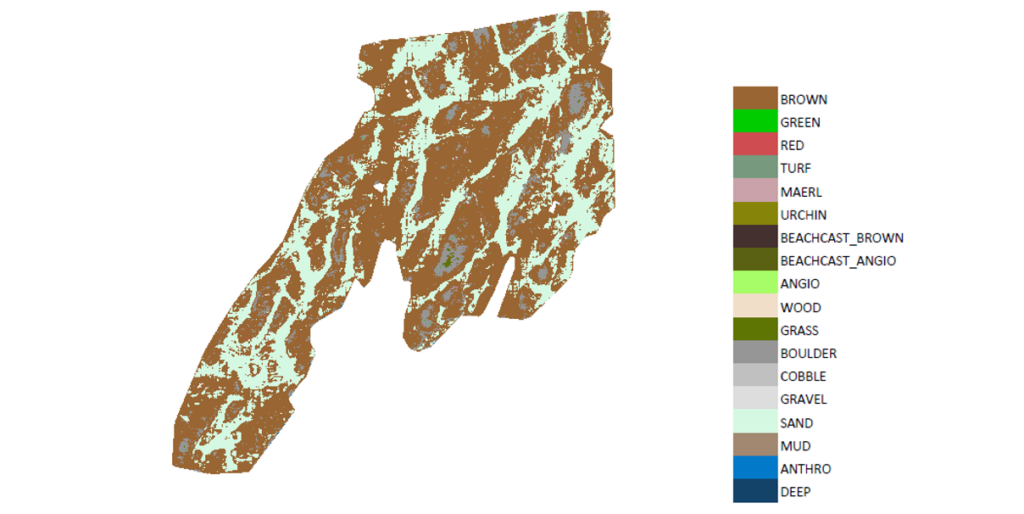
To learn more about this project, please contact:
Project: SeaBee – Norwegian Infrastructure for drone-based research, mapping and monitoring in the coastal zone
Partners: The Norwegian Institute for Water Research (NIVA), the Norwegian University of Science and Technology (NTNU), the Norwegian Institute for Nature Research (NINA), the Institute of Marine Research (IMR) and GRID-Arendal
Funding: The Research Council of Norway
Period: 2020-2024
Further reading: